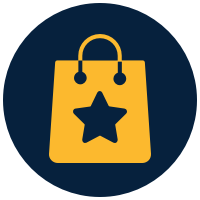
Overview
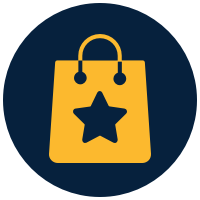
This course covers the learning outcomes and goals of understanding generalization in deep networks, teaching skills such as minimizing classification error and using gradient descent for optimization. The teaching method involves theoretical explanations and examples. The intended audience for this course is individuals interested in deep learning and neural networks.
Syllabus
Intro
Deep Networks can avoid the curse of dimensionality for compositional functions
Minimize classification error minimize surrogate function
Motivation: generalization bounds for regression
GD unconstrained optimization gradient dynamical system
Example: Lagrange multiplier
Explicit norm constraint gives weight normalization
Overparametrized networks fit the data and generalize
Gradient Descent for deep RELU networks
Taught by
MITCBMM